The Georgia COVID-19 updates are freely available to all. If you’d like to support this work financially, then please click the subscribe button below.
Some thoughts on Rt
I’ve been asked a few times about my thoughts on Rt and have given the quick answer that I don’t put much stock in it because it hasn’t been very valuable in predicting trends in this pandemic. But given that our Governor is now using that as a justification for policy decisions in the pandemic, I think it’s probably time to give this a more thorough examination.
First, in the beginning of the pandemic we were discussing the idea of R0, pronounced “arr not.” Yes, I know the “0” and the “t” in Rt is supposed to be subscript but I have limited formatting options here on Substack, so bear with me. But R0 (the basic reproduction number) is an estimate of how many individuals a sick person can infect on average over the course of an outbreak. Because it is an average, there will be people who spread more or less to others. The higher the number the more people a sick person can infect. If the number is higher than 1, then an outbreak is likely to continue. If the value is less than one, then the outbreak is likely to die out.
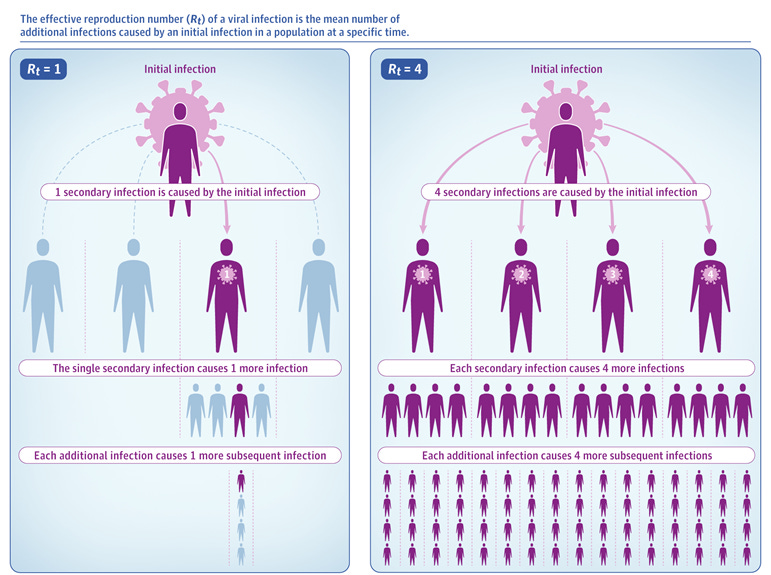
But there is some value in seeing how that value changes over time given that some people will hypothetically develop immunity and interrupt that chain of transmission, or that certain public health interventions will limit the ability of people to spread illness to others. Hence, one can use the effective reproduction number that takes these things into consideration. It, too, is an average and it is a model - meaning it isn’t a hard piece of data like confirmed case counts, but rather an estimate or prediction.
Every model has certain assumptions that should be clearly communicated to the people who use the model. For example, if a state institutes a shelter in place order, one can assume that transmission will go down and then try to quantify that reduction into their model. But once a model’s assumptions are violated (i.e. the shelter in place order is lifted), then the model loses its value and can’t be used to predict trends. Because this is a model, there can be different assumptions baked in, depending on who is doing the modeling. So different groups might report different Rt values and they aren’t necessarily interchangeable because of those differing assumptions.
One of the biggest assumptions for Rt is that natural infection with SARS-CoV-2 (the virus that causes the disease, COVID-19), confers immunity and ideally long-term immunity. But we don’t have a clear idea of whether that is correct or not. We know that people produce antibodies, but we don’t know whether they protect against future infection or for how long. For most infectious diseases that post-recovery immunity does take place, but we have infections where that isn’t the case (such as tetanus). And we don’t have enough time nor data yet to know where COVID-19 falls within that spectrum. For that reason, it might not be correct to assume that people who have previously been infected are “sidelined” from participating in future disease transmission and it might not be appropriate to subtract those individuals out from the Rt models. Another potential snag with Rt assumptions is that it relies on available data for cases, deaths, hospitalizations, etc. And all of that depends on testing. For that reason, countries or states with robust testing are going to produce more reliable Rt values. In other words, the Rt value for a country like South Korea is probably more reliable than for the United States. But without robust testing (or even adequate testing), then the modelers have to make a second assumption about how many people are sick/infected but missed because of the lack of testing or delays in testing. Below I’ve provided some of the limited assumption information that is provided by the popular Rt.live site for estimating Rt, built by the founders of Instagram. Finding their list of assumptions that are factored into their model is not easy for the layperson (this should be red flag number one). But from their FAQ section, they identify some limitations that could impact the validity of their model. It’s noteworthy that all of these issues are going to be impacted by testing output - a problem that Georgia has been plagued with for a long time.
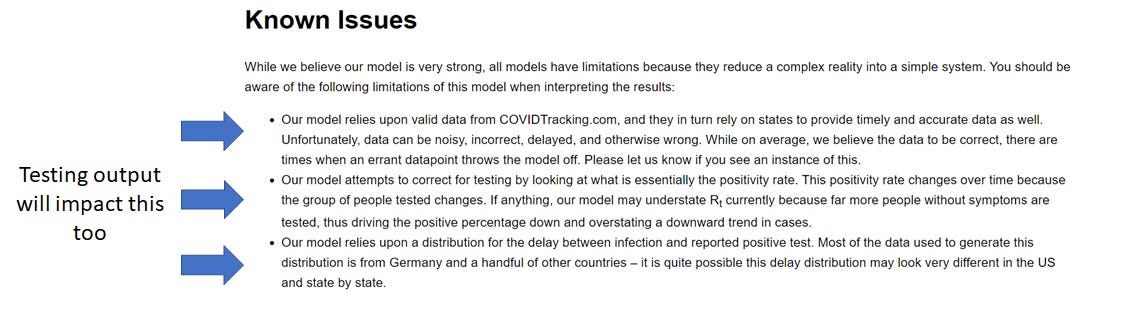
Another model for Rt is provided by the Harvard Chan School of Public Health. I prefer this one for at least two reasons: (1) they clearly lay out their assumptions in the “about” tab and (2) when you’re having to make assumptions about infectious disease, it might be best to have scientists with a background in infectious disease and public health doing that than web developers. To be abundantly clear, I deeply admire the crowd-sourcing spirit of people wanting to help and get involved in combating this pandemic - it is a bright glimmer of community-oriented hope in a time when we desperately need it. But if I have a choice between an auto mechanic versus an accountant to change the oil in my car, I’m always going to choose the mechanic. I think we can appreciate that expertise matters.
Models are great for things like building capacity. For example, let’s say that a model predicts that a surge in cases might be expected in mid-September. Knowing that, we could build testing, contact tracing, and hospital capacity now to prepare. It’s not unlike when a hurricane model shows a potential path and the cities in the cone of uncertainty issue evacuation orders. They can also be used to estimate the risk of different decisions: for example, how many people will get sick if we open schools with a class size of 10 versus 25? But because models are based on assumptions, not all models are equal and there is a lot of uncertainty. I’ve seen some models predict our case surge with surprising accuracy and others that were way off. For that reason, it is problematic to make policy decisions based on them.
But in addition to the problems associated with assumptions within models, generally, the Rt metric hasn’t been terribly effective at predicting what we’ve seen on the ground. Using the Harvard Rt model, let’s look at how Rt compared before, during, and after our surge in cases that began around June 21st. First, let’s orient ourselves. The dashed vertical line represents an Rt value of 1 - the threshold above or below which a disease is increasing or decreasing. So anything to the right means we’re above 1. There’s a time slider on the left to allow you to progress through time. I’ve marked Georgia with a blue block arrow. Another thing to note is the bar for each state. The dot represents the best estimate while the bars extending from it represent the confidence interval. The wider the confidence interval, the less trustworthy the estimate (that’s true for the Rt.live site too). You’ll notice that this is a problem more among states with low population density or low transmission of disease. Next, because of incubation period and delays in testing, cases are detected based on transmission that was happening up to two weeks prior. So I’m starting us out two weeks prior to the onset of the case surge.
Before the surge, but when transmission that triggered it was most likely to be occurring:
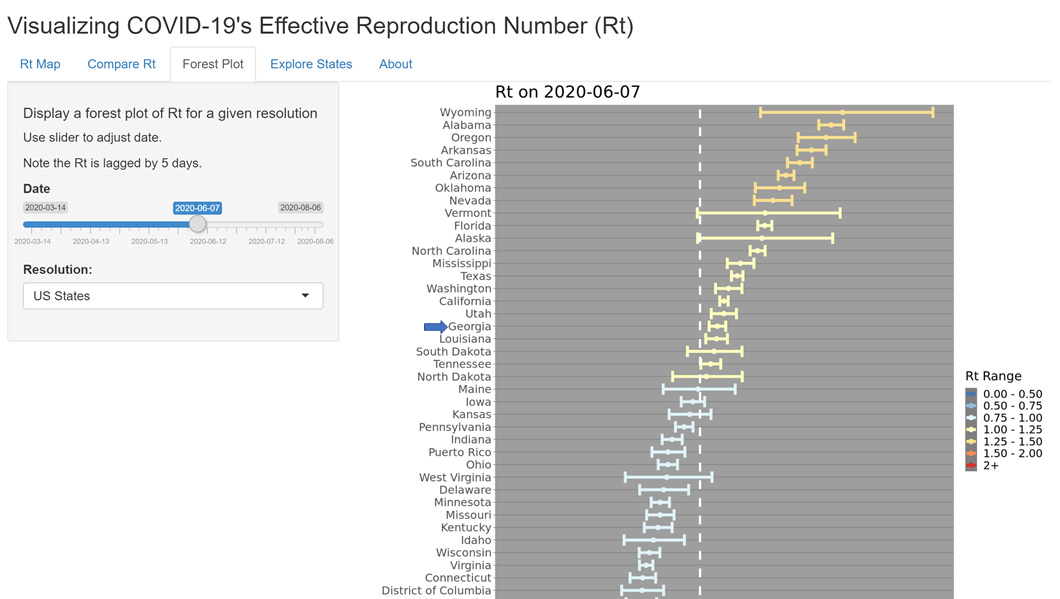
During the height of the surge when hospitals were running out of bed space.
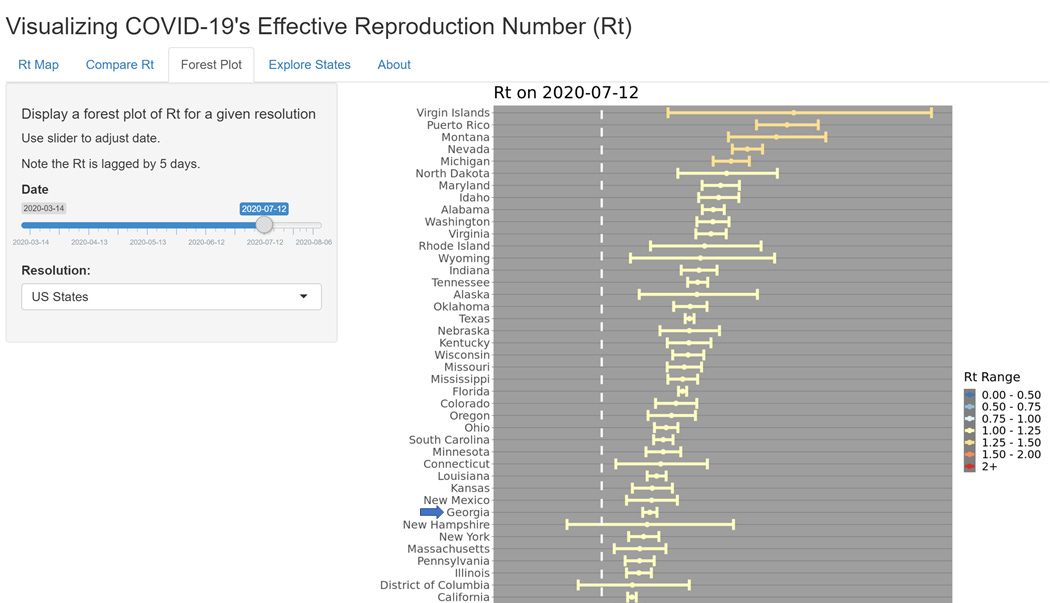
The most recent data, after our case surge allegedly plateaued.
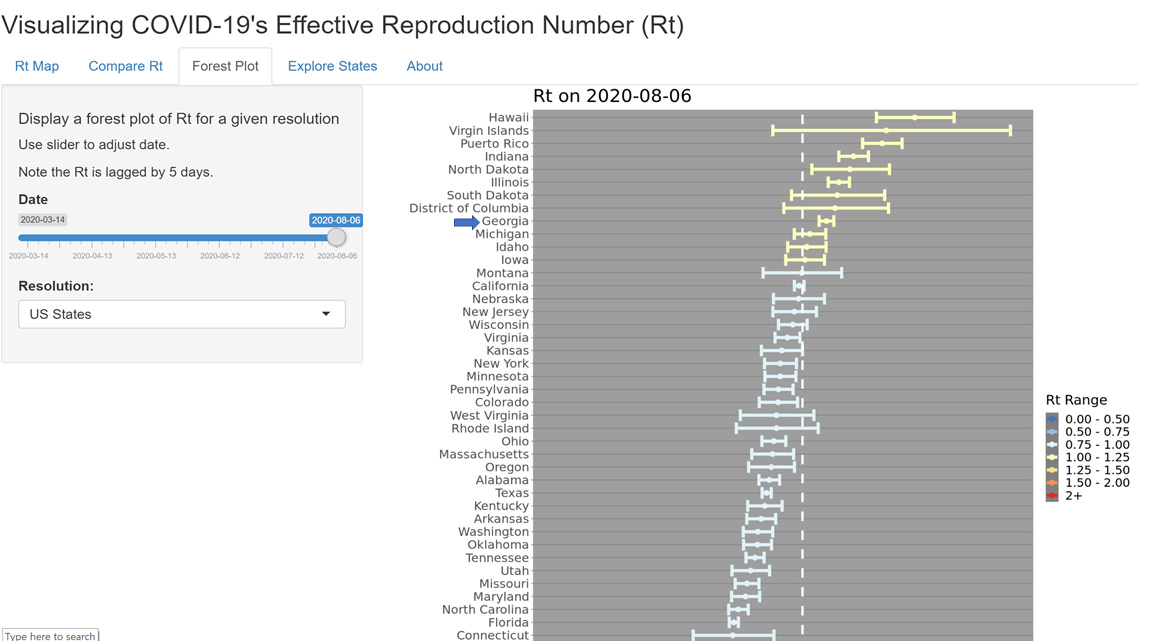
While our ranking among the states has varied over time, our position relative to 1 really hasn’t. We’ve been consistently above 1 the whole time. And we were actually closer to 1 prior to the surge in cases than we are now. If we lined up the Rt ranges for before the surge and now, just for Georgia, the confidence intervals would likely overlap meaning that they are statistically not that different from each other. Does that mean we have another surge on the horizon?
Going back to the Rt model from the Rt.live, the model also doesn’t match well with what we experienced. Our earlier case peak in April was growing during a time that Rt would have predicted that disease transmission was low. On the flip side, when the state reopened and Rt was above 1, it predicted an increase in transmission that we didn’t see materialize in a surge until late June.
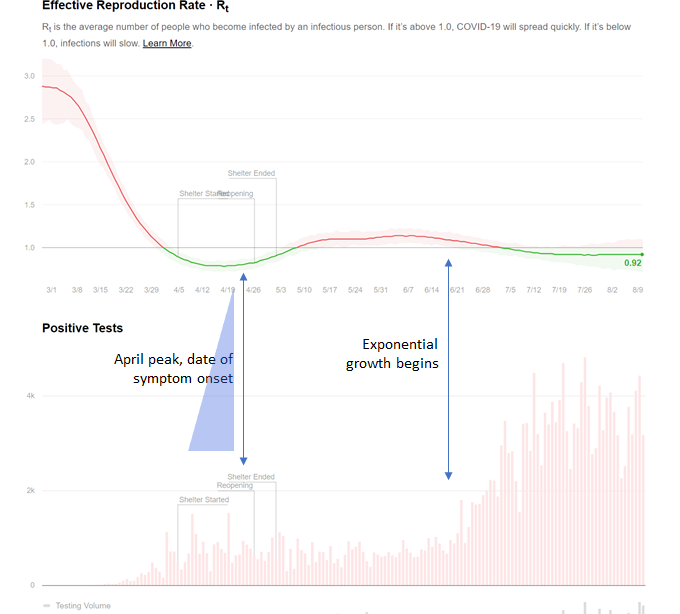
Moreover, the variation we see over time around the threshold of 1 just looks like the statistical range of error. In summary, it just doesn’t seem to mean anything.
What I and others have noticed, though, is that while people who work in public health do not put a lot of faith in Rt (for all the reasons indicated above), politicians and their followers do. I know there’s a desire among all of these data to just have one barometer to gauge how we’re doing. But infectious disease, and a pandemic especially, isn’t that simple. And I think we’d all like to celebrate good news right now. But if an Rt model is predicting that we are reducing the rate of disease right now when we just reopened schools (and hence a new opportunity for widespread disease transmission) and at a time when tests are taking up to three weeks to come back, then it is probably not a very good predictive model. So if someone is hanging their hat on an overly optimistic model of Rt (like the one from Rt.live), it probably shows they don’t have a strong background in infectious disease or public health…at a time when a scientific problem needs scientific analysis. To summarize, Rt is not a value that I check daily, weekly or even monthly. Every time I do check it, I’m disappointed because it doesn’t predict nor reflect our situation on the ground. But if I were, I would be finding the best model by the most qualified individuals to get the best estimate.
Testing
Today the Georgia Department of Public Health reports 21,257 new test results, a below average day for us based on the last two weeks’ trend. Of those, 11% were positive. That’s higher than we’ve seen in the past four days, but it is on track with where we were a week ago. Below is a look at how today’s newly reported cases were backdated on the case curve by date of symptom onset (see the blue bars along the bottom). The image is hard to read. But the graphic is linked to the source, so I would encourage you to visit and explore.
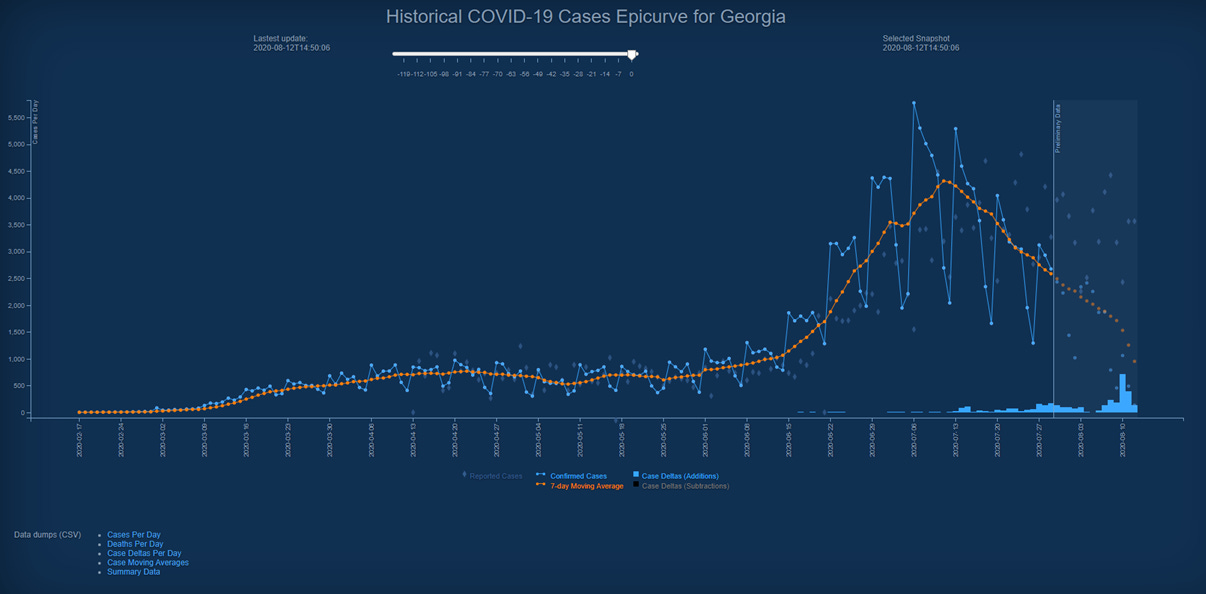
As expected, most of the newly identified cases are within the 14 day window of uncertainty. But a surprising number, 1224, were backdated prior to the 14-day threshold. That’s 34.3% of the net increase in cases today (3565) relative to yesterday. It goes to show how our testing backlogs are impacting both disease reporting and ultimately contact tracing.
Cases
There is a net increase of 3565 cases today. Again, in the “new today” section of the DPH report, they are reporting the data prior to de-duplication and correction. I think that’s probably a good-faith effort at transparency, but it is very confusing in the meantime. But today’s case total is 226,153 and yesterday’s was 222,588. So 226,153 - 222,588 = 3565 net increase.
Here’s where the case increases have been most intense over the past two weeks, adjusted for population size.
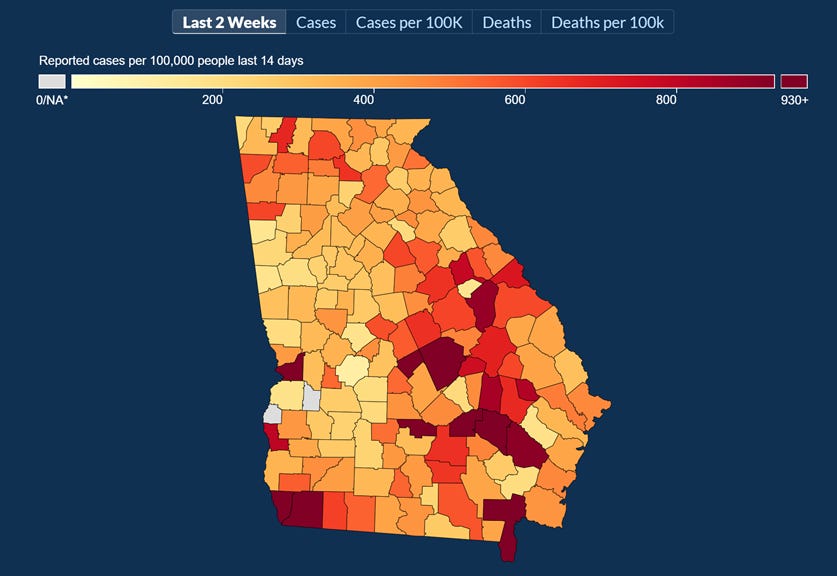
It is not shocking but nonetheless troubling to see that the area in Hospital Region H is filled with darkened counties. They have been plagued by limited critical care bed capacity throughout the pandemic, but especially so in the past three weeks. In fact, it has led the city of Dublin (Laurens County) to institute a mask mandate and to ask the governor for a statewide mandate.
Of today’s newly reported cases, 35% came from nonrural counties not part of the Atlanta metro, 29% came from rural counties. In other words, two thirds of the cases are from outside the Atlanta metro.
Hospitalizations
Current hospitalizations appear to have stabilized over the past three weeks.
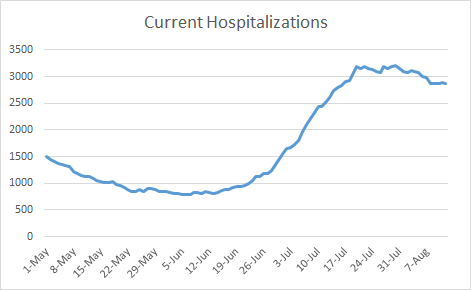
New hospital admissions are more variable, but the trend seems to have decreased relative to where it was last week (a good thing!).
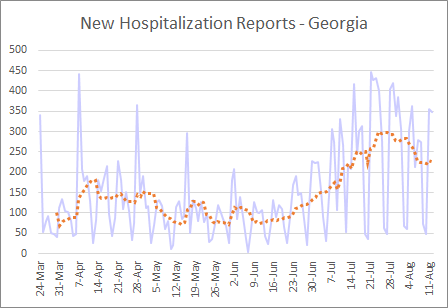
Georgia Emergency Management Agency has been experiencing some delays (and one interruption entirely) in reporting the hospital capacity data over the past week or so. But based on yesterday’s report, here’s how the ICU bed capacity compared across hospital regions.
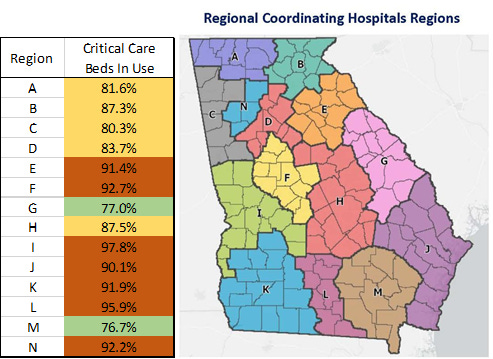
Deaths
It was another grim day for deaths, with a net increase of 105 compared to yesterday’s total. Below, you can see how today’s newly reported deaths were backdated on the date of death graph (see the red bars at the bottom).
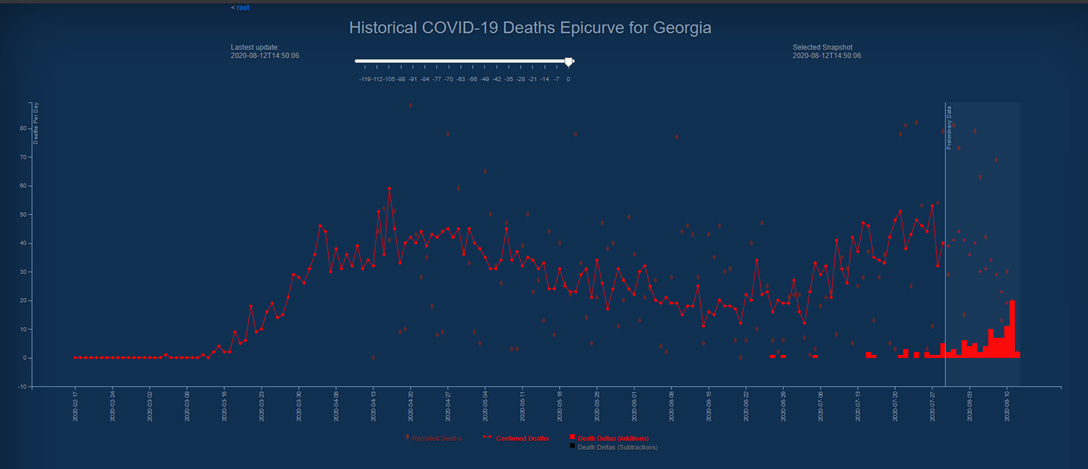
The new statewide total is 4456. Forty of today’s deaths came from nonrural counties outside of the Atlanta metro and the next highest county type was 33 from rural counties. In other words, 73% of the deaths reported today were from outside of the Atlanta metro.
References
https://dph.georgia.gov/covid-19-daily-status-report
https://jamanetwork.com/journals/jama/fullarticle/2765665
https://rt.live/
http://metrics.covid19-analysis.org/
http://208.97.140.204:8080/epicurveInteractive-cases.html
https://www.medpagetoday.com/infectiousdisease/covid19/86171
https://www.nature.com/articles/d41586-020-02009-w
https://41nbc.com/2020/08/12/city-of-dublin-passes-mask-mandate/#:~:text=The%20ordinance%20passed%20in%20a,under%20the%20age%20of%2010.